The Impact of Training Costs on AI Model Development
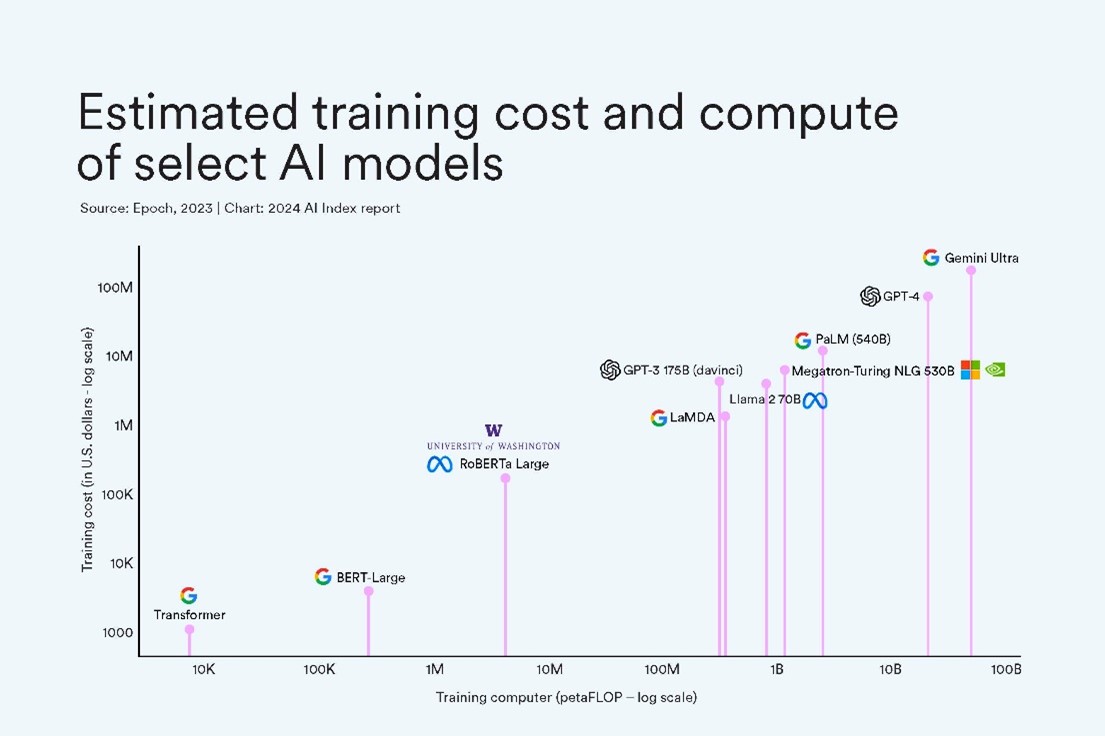
Edge Computing as a Key Development Area
Performance Gaps in AI Development
Global Leadership in AI Models
Financial Implications of AI Training Costs
Shift Towards Cost-Effective Small Models
AI Trends and Insights
The latest AI Index Report 2024, released by Stanford University's Human-Centered Artificial Intelligence on April 15, 2024, provides comprehensive insights into AI trends and developments. The report highlights the rapid advancements in AI, emphasizing the performance of AI in various benchmarks such as image classification, visual reasoning, and English understanding.
Edge Computing as a Key Development Area
Edge computing is highlighted as a crucial area for future development, particularly for small and medium-sized companies. This technology serves as an important link between AI and these companies, facilitating their growth and development by enabling more localized processing and reducing the reliance on centralized data centers.
Performance Gaps in AI Development
While AI has surpassed human performance in several benchmarks, it still lags in complex tasks like competition-level mathematics, visual common-sense reasoning, and planning. This lag is attributed to the limitations of the mainstream model structure, which predominantly utilizes transformer architectures.
Global Leadership in AI Models
The United States leads in the creation of top AI models, with 61 prominent models originating from US institutions in 2023. This far outpaces the European Union’s 21 and China’s 15 models. The report notes that 72% of these models come from the industry sector, underscoring the rapid development driven by companies like Google and OpenAI. However, the exponential growth in training costs is sidelining academia and government efforts in AI development.
Financial Implications of AI Training Costs
The Financial Times has detailed the significant financial burden associated with training large AI models. For instance, Google's Gemini Ultra and OpenAI’s GPT-4 have training costs estimated at $191 million and $78 million, respectively. The cost disparity in generating responses highlights the immense financial considerations involved in AI development and data management.
Shift Towards Cost-Effective Small Models
In response to high training costs and operational complexities, large tech companies are developing cost-effective small models. These smaller models, such as Microsoft's phi-3 mini and Meta's Llama3, contain fewer parameters yet perform comparably to larger models. This trend allows for localized AI applications, addressing privacy concerns and reducing operational costs.
Future of Chip Development
TECHSPOT analysts predict that future chip development will be heavily influenced by the needs of AI model training and deployment. By 2024, it is expected that 15% of chips will be dedicated to training models, 45% will be used in data centers, and 40% will support edge computing scenarios, reflecting the growing importance of small models in the AI landscape.
FAQ
- Q: What is the primary reason for the high cost of training large AI models?
- A: The primary reason for the high cost of training large AI models is the extensive computational resources required, which leads to exponentially growing expenses. For example, Google's Gemini Ultra training costs are estimated at $191 million, and OpenAI's GPT-4 costs are around $78 million.
- Q: How are large tech companies addressing the high costs and operational complexities of AI model training?
- A: Large tech companies are addressing these challenges by developing cost-effective small models that require fewer computational resources and are easier to manage. Examples include Microsoft's phi-3 mini and Meta's Llama3, which offer robust performance while reducing training and operational costs.
- Q: What role will edge computing play in the future development of AI, according to the AI Index Report 2024?
- A: According to the AI Index Report 2024, edge computing will play a crucial role in future AI development, particularly for small and medium-sized companies. It enables localized data processing, reduces dependency on centralized data centers, and supports the deployment of cost-effective small models, thereby enhancing accessibility and efficiency in AI applications.